UBIQUITOUS
Project informationen
- Funded by DFG
- In cooporation with Chair of Econometrics and Statistics, esp. in the Transport Sector
- Duration of 3 years, started in August 2019
An efficient air transport system requires both individually locally and flow based globally optimized aircraft trajectories. However, in commercial air transportation, aircraft trajectories have to comply with a variety of legal requirements as declared in the Implementation Rules for Flight Operations (IR-OPS1) covering e.g. minimum fuel requirements for a given flight mission leading to aircraft mass constraints, or lateral path restrictions based on worldwide published available waypoints according to ICAO AIRAC2 and limited number of available cruising pressure altitudes.
An optimization further depends on the network of supply and demand, the available fleet, political decisions and the weather predictability as main input factor for trajectory optimization. This information can be extracted by ADS-B (Automatic dependent surveillance—broadcast) data.
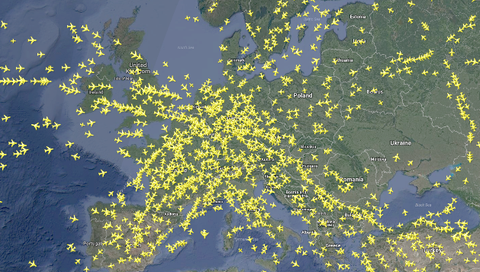
European air traffic network structure
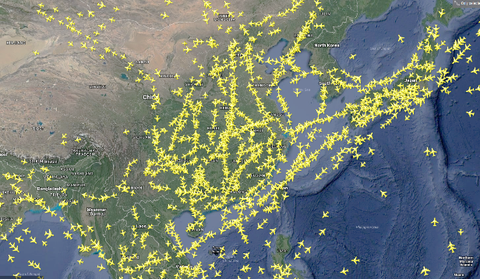
Chinese air traffic network structure
Thanks to ICAO's mandate on aircraft ADS-B installation and free accessible data community like Opensky Network, open flight track data including position, altitude, velocity and vertical speed of commercial aircraft has worldwide become available. These data provide an alternative source for modeling aircraft performance while considering environmental unpredictability. Some literature already explores trajectories clustering and predicting using machine learning methods regarding environmental constraints and uncertainties. However, it remains a challenge to analyze data not only with enormous volume but also generating at a considerable velocity. Conclusive results are not yet fully yielded.
The research project "UBIQUITOUS" aims to study and recognize patterns of historical trajectories in ADS-B data. Using identified features, this research proceeds to improve classification of ATM (Air Traffic Management) structure, airline network, routing structure and fleet scheduling behaviors. With better understanding of traffic pattern, upgraded trajectories regarding cost, fuel and climate impact are supposed to be suggested using data driven approaches.
Unsupervised learning methods such as K-means, K-nearest neighbor and hierarchical clustering can be used to investigate clustering of single trajectories. For airline network structures, graphic network models could be applied to do comparative analysis about patterns of graph in China and Europe. Besides descriptive analysis, traditional statistical models as regression and machine learning methods like Support vector machine regression, decision tree regression and neural network regression can model improved trajectory forecasting.
Ansprechpartner
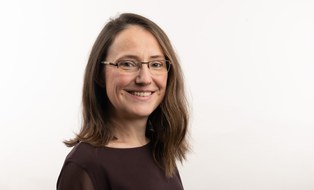
Research Associate
NameDr.-Ing. habil. Judith Rosenow
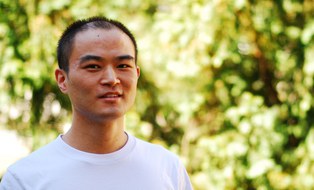
Wissenschaftlicher Mitarbeiter
NameMr Gong Chen M.Sc.